The world we live in is rapidly evolving, with technological advancements reshaping the way we interact with our environment and each other. At the forefront of this transformation are the convergence of the Internet of Things (IoT) and Artificial Intelligence (AI), two powerful technologies that are poised to redefine the future of connectivity.
Introduction
In the era of the digital revolution, the integration of IoT and AI has emerged as a crucial driver of innovation, presenting unprecedented opportunities for enhancing our daily lives, streamlining business operations, and addressing global challenges. This blog post will delve into the fascinating intersection of these two technologies, exploring their individual landscapes, the current state of their integration, the benefits and challenges of their combined application, and the exciting future possibilities that lie ahead.
Overview of IoT (Internet of Things)

The Internet of Things (IoT) is a rapidly growing field that encompasses a network of interconnected devices, sensors, and systems that can collect, exchange, and analyze data. This vast ecosystem of connected devices, ranging from smart home appliances to industrial equipment, has the potential to transform the way we live, work, and interact with the world around us.
The Scope of IoT
- Smart Homes: IoT devices in the home, such as smart thermostats, lighting systems, and security cameras, enable remote control, automation, and optimization of household functions.
- Smart Cities: IoT-powered infrastructure, including traffic management systems, waste management, and public service monitoring, aims to improve efficiency and quality of life in urban environments.
- Industrial IoT (IIoT): IoT applications in the industrial sector, such as predictive maintenance, supply chain optimization, and energy management, drive increased productivity and cost savings.
- Wearable Devices: IoT-enabled wearables, like fitness trackers and smartwatches, provide real-time data on health, activity, and personal well-being.
Key Enablers of IoT
- Connectivity: The widespread adoption of technologies, such as Wi-Fi, Bluetooth, and cellular networks, has enabled seamless connectivity between IoT devices and the internet.
- Sensors: Advancements in sensor technology have led to the development of smaller, more accurate, and energy-efficient sensors that can be embedded into a wide range of devices.
- Cloud Computing: The availability of robust cloud infrastructure has facilitated the storage, processing, and analysis of the vast amounts of data generated by IoT devices.
- Edge Computing: The emergence of edge computing, which processes data closer to the source, has improved response times and reduced the reliance on cloud-based processing.
Challenges in IoT Adoption
- Security and Privacy Concerns: The interconnected nature of IoT devices raises concerns about data privacy and the potential for cyber-attacks, which must be addressed through robust security measures.
- Interoperability and Standardization: The lack of universal standards and protocols across different IoT ecosystems can hinder seamless integration and data exchange between devices.
- Data Management and Analytics: The sheer volume of data generated by IoT devices presents challenges in terms of storage, processing, and extracting meaningful insights.
- Power Consumption and Battery Life: Ensuring efficient power management and extended battery life for IoT devices is crucial for their widespread adoption and deployment.
Overview of AI (Artificial Intelligence)
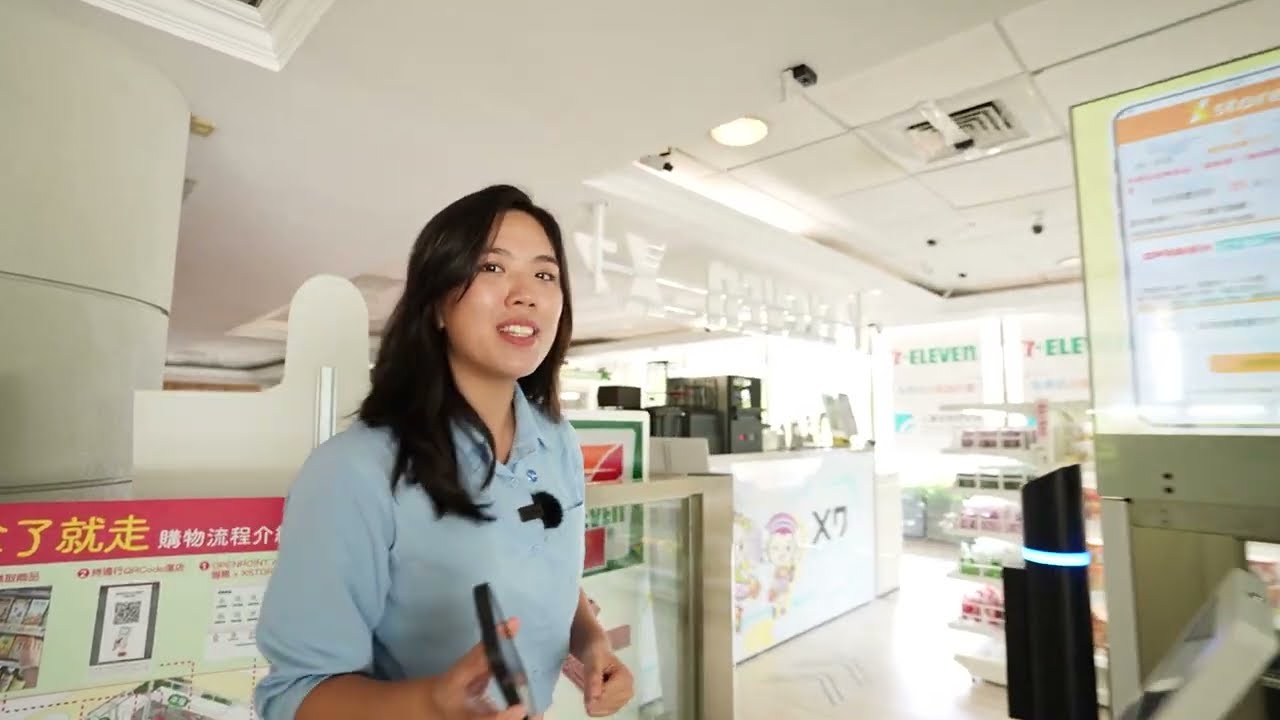
Artificial Intelligence (AI) is a field of study that focuses on the development of systems and machines capable of performing tasks that typically require human intelligence, such as learning, problem-solving, decision-making, and perception.
The Scope of AI
- Machine Learning: This subfield of AI involves the development of algorithms and statistical models that enable systems to learn and improve from data without being explicitly programmed.
- Natural Language Processing (NLP): NLP focuses on the interaction between computers and human language, enabling machines to understand, interpret, and generate human-like text or speech.
- Computer Vision: This area of AI deals with the ability of machines to interpret and understand digital images and videos, allowing for applications like object recognition, image classification, and scene understanding.
- Robotics: AI-powered robotics combines sensors, actuators, and intelligent control systems to create autonomous or semi-autonomous machines that can perform a wide range of physical tasks.
Key Enablers of AI
- Increased Computing Power: Advancements in hardware, such as powerful processors and GPUs, have significantly enhanced the computational capabilities required for complex AI algorithms.
- Big Data: The availability of vast amounts of data, from various sources, has provided the necessary fuel for training and improving AI models.
- Advancements in Algorithms: Continuous improvements in machine learning algorithms, such as deep learning and reinforcement learning, have led to significant breakthroughs in AI capabilities.
- Cloud Computing and Storage: Cloud-based infrastructure has enabled the scalable deployment and accessibility of AI-powered applications and services.
Challenges in AI Adoption
- Ethical Concerns: The increasing use of AI in decision-making processes raises concerns about bias, transparency, and the potential for unintended consequences.
- Interpretability and Explainability: The complexity of some AI models, particularly in deep learning, can make it challenging to understand and explain the reasoning behind their decisions.
- Data Availability and Quality: The success of AI systems is heavily dependent on the availability and quality of the data used for training, which can be a significant challenge in certain domains.
- Talent and Skill Gap: The rapid advancements in AI have created a shortage of skilled professionals, making it challenging for organizations to build and maintain effective AI-powered solutions.
Current Intersection of IoT and AI
The convergence of IoT and AI has been a transformative force, unlocking new possibilities and reshaping various industries. By integrating these two powerful technologies, organizations can leverage the wealth of data generated by IoT devices and apply advanced AI-driven analytics to drive unprecedented insights and decision-making.
IoT-Powered Data Collection
- Sensor Data: IoT devices equipped with sensors can collect a vast array of data, ranging from environmental conditions to equipment performance, providing a rich data source for AI-driven analysis.
- Real-Time Monitoring: IoT systems enable continuous, real-time monitoring of various processes and activities, allowing for immediate detection of anomalies or changes that can be analyzed by AI algorithms.
- Increased Connectivity: The interconnectivity of IoT devices facilitates the aggregation and centralization of data, making it more accessible for AI-powered processing and decision-making.
AI-Driven Data Analysis
- Predictive Maintenance: By analyzing sensor data from IoT-enabled industrial equipment, AI algorithms can identify patterns and predict potential failures, enabling proactive maintenance and reducing downtime.
- Automated Decision-Making: AI models can process the data collected by IoT devices and make autonomous decisions, such as adjusting energy consumption in smart homes or optimizing supply chain operations.
- Personalization and Optimization: AI-powered IoT systems can adapt and personalize their behavior based on individual user preferences and habits, enhancing the overall user experience.
Improved Efficiency and Optimization
- Resource Optimization: The integration of IoT and AI can lead to more efficient utilization of resources, such as energy, water, and materials, by identifying patterns and optimizing consumption.
- Operational Efficiency: AI-driven analysis of IoT data can help streamline business processes, improve productivity, and reduce operational costs across various industries.
- Enhanced Customer Experience: The combination of IoT and AI enables the development of more personalized and responsive customer experiences, tailored to individual needs and preferences.
Enhanced Situational Awareness
- Anomaly Detection: AI algorithms can analyze IoT sensor data to identify anomalies or deviations from normal patterns, enabling early detection and prevention of issues.
- Automated Responses: IoT systems integrated with AI can quickly respond to changing conditions or emergencies, such as adjusting building systems during a natural disaster or triggering safety protocols in industrial environments.
- Predictive Insights: By leveraging the data collected by IoT devices and applying advanced AI techniques, organizations can gain valuable predictive insights to make more informed decisions and anticipate future trends.
Benefits of Combining IoT and AI
The synergistic integration of IoT and AI offers a wealth of benefits, transforming various industries and driving innovation across a wide range of applications.
Improved Decision-Making
- Data-Driven Insights: The combination of IoT data collection and AI-powered analytics enables organizations to gain deeper, data-driven insights that can inform more strategic and informed decision-making.
- Automated Decision-Making: AI algorithms can process real-time IoT data and make autonomous decisions, reducing the need for manual intervention and improving the speed and efficiency of decision-making processes.
- Adaptive and Personalized Solutions: AI-powered IoT systems can adapt and personalize their responses based on individual user preferences or changing environmental conditions, leading to more tailored and effective solutions.
Enhanced Operational Efficiency
- Predictive Maintenance: By analyzing IoT sensor data, AI algorithms can predict equipment failures or performance issues, enabling proactive maintenance and reducing costly downtime.
- Supply Chain Optimization: The integration of IoT and AI can help optimize supply chain processes, from inventory management to logistics and distribution, leading to improved efficiency and cost savings.
- Energy and Resource Management: IoT-enabled monitoring and AI-driven optimization can help organizations better manage their energy consumption, water usage, and other resources, leading to significant cost savings and environmental benefits.
Improved Safety and Compliance
- Anomaly Detection and Incident Response: AI-powered IoT systems can quickly detect anomalies or safety incidents and trigger automated responses, enhancing safety and reducing the risk of accidents or disasters.
- Regulatory Compliance: The data collected by IoT devices and the insights generated by AI can help organizations better monitor and adhere to various industry regulations and standards.
- Environmental Sustainability: The optimization of resource usage and the ability to predict and respond to environmental changes can contribute to more sustainable and eco-friendly practices.
Enhanced User Experience
- Personalization and Customization: The integration of IoT and AI allows for the development of highly personalized and customized user experiences, tailored to individual preferences and needs.
- Seamless Automation: IoT-powered automation, combined with AI-driven decision-making, can create a more seamless and effortless user experience, reducing the need for manual intervention.
- Predictive Capabilities: AI-powered IoT systems can anticipate user needs and preferences, proactively providing recommendations or adjusting their behavior to enhance the overall user experience.
Challenges of Integrating IoT and AI
While the combination of IoT and AI holds immense potential, there are also several challenges that organizations must address to ensure successful integration and deployment.
Interoperability and Standardization
- Diverse IoT Ecosystems: The lack of standardized protocols and communication interfaces across different IoT devices and platforms can hinder seamless integration and data exchange.
- Fragmented AI Landscape: The wide range of AI tools, frameworks, and deployment models can make it challenging to ensure compatibility and seamless integration with IoT systems.
- Collaborative Efforts: Overcoming these interoperability challenges often requires collaborative efforts among IoT and AI vendors, industry associations, and regulatory bodies to establish common standards and protocols.
Data Management and Security
- Data Volume and Variety: The massive amount of data generated by IoT devices, along with the diversity of data types, can overwhelm traditional data management and storage solutions.
- Data Quality and Preprocessing: Ensuring the accuracy, completeness, and reliability of IoT data is crucial for effective AI-driven analysis, which often requires significant data preprocessing and cleansing efforts.
- Cybersecurity Risks: The interconnected nature of IoT and AI systems can expose them to various cybersecurity threats, such as data breaches, malware, and unauthorized access, which must be addressed through robust security measures.
Talent and Skill Gap
- Specialized Expertise: Integrating IoT and AI requires a unique blend of skills, including hardware engineering, software development, data science, and domain-specific knowledge, which can be challenging to find and retain.
- Upskilling and Training: Organizations must invest in upskilling and training their workforce to ensure they have the necessary skills to design, deploy, and maintain IoT-AI solutions effectively.
- Collaborative Partnerships: Bridging the talent and skill gap may also involve forming strategic partnerships with specialized service providers or research institutions to access the required expertise.
Ethical and Regulatory Concerns
- Bias and Transparency: AI-driven decision-making in IoT systems raises concerns about algorithmic bias and the need for greater transparency in how these decisions are made.
- Privacy and Data Governance: The collection and use of personal data by IoT devices, combined with AI-powered analysis, require robust privacy policies and data governance frameworks to protect individual rights and ensure compliance with regulations.
- Regulatory Landscape: As the IoT-AI landscape continues to evolve, organizations must stay informed about changing regulations and industry standards to ensure their solutions comply with relevant laws and guidelines.
Future Possibilities and Trends
The synergistic integration of IoT and AI holds immense promise, and the future of this union is filled with exciting possibilities that will continue to transform various industries and our daily lives.
Advancements in Edge Computing
- Distributed Intelligence: The rise of edge computing, where data processing and analysis occur closer to the source, will enable IoT devices to make more autonomous, real-time decisions without relying solely on cloud-based AI.
- Reduced Latency: Edge computing will help minimize latency in IoT-AI systems, enabling faster response times and critical applications, such as autonomous vehicles and real-time industrial control.
- Enhanced Privacy and Security: Performing AI-driven analysis at the edge can help reduce the exposure of sensitive data to external networks, improving privacy and security.
Proliferation of Intelligent Sensors
- Sensor Fusion and Multi-Modal Sensing: IoT devices will incorporate a broader range of sensors, enabling the collection of richer, multi-modal data that can be analyzed by advanced AI algorithms.
- Miniaturization and Embedded AI: Continued advancements in sensor technology and the integration of AI capabilities directly into IoT devices will lead to smaller, more powerful, and energy-efficient smart sensors.
- Autonomous Decision-Making: IoT sensors with embedded AI will be able to make localized decisions, reducing the need for cloud-based processing and enabling faster responses to changing conditions.
Advancements in AI Algorithms
- Explainable AI: As AI systems become more complex, there will be a growing emphasis on developing algorithms that can provide more transparent and interpretable decision-making processes.
- Reinforcement Learning: AI-powered IoT systems will leverage reinforcement learning techniques to continuously adapt and optimize their performance based on feedback and environmental changes.
- Federated Learning: The integration of federated learning, where AI models are trained across multiple IoT devices without centralizing data, will enhance privacy and enable more personalized AI-driven applications.
Increased Automation and Autonomy
- Autonomous Vehicles and Drones: The combination of IoT sensors and AI-powered decision-making will drive the development of autonomous vehicles and unmanned aerial vehicles (UAVs) for various applications, such as transportation, delivery, and surveillance.
- Smart Factories and Industry 4.0: The integration of IoT and AI will transform industrial environments, enabling fully automated, data-driven, and self-optimizing “smart factories” that can adapt to changing production needs.
- Intelligent Infrastructure: IoT-AI systems will be increasingly integrated into urban infrastructure, such as traffic management systems, energy grids, and water distribution networks, to create more efficient and responsive “smart cities.”
Personalized and Adaptive Services
- Intelligent Home and Building Systems: IoT-powered homes and buildings will leverage AI to create personalized environments that adapt to the preferences and needs of their occupants, enhancing comfort, energy efficiency, and overall user experience.
- Predictive Healthcare: The integration of IoT devices, such as wearables and medical sensors, with AI-driven analytics will enable more proactive and personalized healthcare, allowing for early disease detection, preventive measures, and customized treatment plans.
- Adaptive Retail and Customer Experiences: AI-powered IoT systems will transform the retail industry, offering personalized product recommendations, dynamic pricing, and seamless omnichannel experiences tailored to individual customer preferences.
Case Studies of Successful IoT and AI Integration
Smart City Initiatives
City of Barcelona, Spain
- Overview: The city of Barcelona has been at the forefront of smart city initiatives, integrating IoT and AI technologies to improve urban efficiency and quality of life.
- Key Initiatives: Barcelona has deployed IoT sensors throughout the city to monitor air quality, traffic congestion, and waste management. AI-powered analytics are used to optimize street lighting, manage parking, and predict maintenance needs for infrastructure.
- Benefits: The integration of IoT and AI has led to significant improvements in energy efficiency, reduced greenhouse gas emissions, and improved service delivery for the city’s residents.
Industrial IoT (IIoT) Applications
Siemens MindSphere
- Overview: Siemens’ MindSphere is an industrial IoT platform that combines IoT data collection with AI-driven analytics to enhance operational efficiency and productivity in manufacturing and industrial environments.
- Key Features: MindSphere leverages IoT sensors to gather real-time data from production equipment, which is then analyzed by AI algorithms to predict equipment failures, optimize maintenance schedules, and improve overall equipment effectiveness.
Introduction
In the rapidly evolving landscape of technology, the convergence of IoT (Internet of Things) and AI (Artificial Intelligence) has emerged as a powerful force driving innovation across various industries. This article delves into the synergistic relationship between IoT and AI, exploring their current intersection, benefits, challenges, future possibilities, and real-world case studies. By combining the vast network of connected devices in IoT with the intelligence and learning capabilities of AI, organizations can unlock new opportunities for automation, optimization, and decision-making.
Overview of IoT (Internet of Things)
IoT refers to the interconnected network of physical devices embedded with sensors, software, and other technologies that enable them to collect and exchange data over the internet. These devices span a wide range of applications, from smart home appliances and wearable devices to industrial machinery and city infrastructure. The key components of an IoT system include sensors to gather data, connectivity for data transmission, cloud platforms for storage and processing, and user interfaces for interaction.
- Sensors: IoT devices are equipped with various sensors, such as temperature, light, motion, and proximity sensors, to capture real-time data about their surroundings.
- Connectivity: IoT devices use different communication protocols, including Wi-Fi, Bluetooth, Zigbee, and cellular networks, to transmit data to centralized servers or other devices.
- Cloud Platforms: Data collected by IoT devices is often stored and processed in cloud-based platforms, where advanced analytics and AI algorithms can derive meaningful insights.
- User Interfaces: To interact with IoT systems, users can access dashboards, mobile apps, or voice assistants that provide information, alerts, and controls for connected devices.
Overview of AI (Artificial Intelligence)
AI encompasses a broad set of technologies that enable machines to perform tasks that typically require human intelligence, such as reasoning, learning, perception, and problem-solving. Machine learning, a subset of AI, focuses on developing algorithms that allow systems to learn from data, identify patterns, and make predictions without explicit programming. Deep learning, a more advanced form of machine learning, involves neural networks with multiple layers that can analyze complex data and extract intricate patterns.
- Machine Learning: AI systems leverage machine learning algorithms to analyze data, learn from patterns, and make decisions or predictions based on new inputs.
- Deep Learning: Deep learning models, inspired by the structure of the human brain, use neural networks to process vast amounts of data and extract hierarchical representations.
- Natural Language Processing (NLP): NLP enables computers to understand, interpret, and generate human language, allowing for applications like chatbots, language translation, and sentiment analysis.
- Computer Vision: AI-powered computer vision algorithms can analyze and interpret visual information from images or videos, enabling applications in object recognition, image classification, and autonomous vehicles.
Current Intersection of IoT and AI
The intersection of IoT and AI is reshaping industries by enabling intelligent automation, predictive analytics, and personalized experiences. IoT devices generate massive volumes of data that AI algorithms can analyze to uncover insights, detect anomalies, and optimize processes in real time. By embedding AI capabilities into IoT devices or integrating IoT data into AI models, organizations can enhance the efficiency, agility, and intelligence of their systems.
- Real-Time Insights: AI algorithms can process streaming IoT data to provide instant insights and actionable recommendations for businesses or consumers.
- Predictive Maintenance: By analyzing IoT sensor data with AI, organizations can predict equipment failures, schedule maintenance proactively, and minimize downtime.
- Smart Decision-Making: Combining IoT sensor data with AI analytics enables systems to make autonomous decisions, adapt to changing conditions, and optimize performance continuously.
- Personalized Experiences: AI-driven IoT applications can personalize user interactions, tailor recommendations, and anticipate needs based on individual preferences or behavior.
Benefits of Combining IoT and AI
The integration of IoT and AI offers a myriad of benefits across industries, revolutionizing processes, services, and experiences. By harnessing the collective power of connected devices and intelligent algorithms, organizations can achieve new levels of efficiency, productivity, and innovation. Some key benefits of combining IoT and AI include improved decision-making, enhanced operational efficiency, predictive capabilities, and personalized services.
- Improved Decision-Making: AI algorithms analyze IoT data to provide valuable insights, trends, and patterns that support strategic decision-making and drive business growth.
- Enhanced Operational Efficiency: IoT devices connected to AI systems can automate routine tasks, optimize workflows, and streamline operations for greater efficiency.
- Predictive Capabilities: AI-powered predictive analytics applied to IoT data enable organizations to forecast trends, anticipate risks, and preemptively address issues before they escalate.
- Personalized Services: By leveraging AI to interpret IoT data, businesses can offer personalized recommendations, tailored services, and customized experiences to meet individual needs and preferences.
Challenges of Integrating IoT and AI
Despite the tremendous potential of combining IoT and AI, several challenges must be addressed to facilitate seamless integration and maximize the value of these technologies. From technical complexities and security concerns to ethical considerations and skill gaps, organizations need to navigate various obstacles to ensure successful deployment and adoption of IoT-AI solutions. Addressing these challenges requires strategic planning, robust frameworks, and continuous innovation to drive sustainable outcomes.
- Interoperability: Ensuring compatibility and seamless communication between diverse IoT devices and AI systems can be challenging due to differing standards and protocols.
- Security Vulnerabilities: Protecting IoT devices from cyber threats and safeguarding AI models from adversarial attacks are critical for maintaining data integrity and system reliability.
- Data Privacy: IoT devices collect vast amounts of personal data, raising concerns about privacy, consent, and compliance with regulations like GDPR and CCPA.
- Complex Algorithms: Developing and optimizing AI algorithms for IoT applications requires specialized expertise, computational resources, and ongoing calibration.
Future Possibilities and Trends
The synergistic integration of IoT and AI holds immense promise, ushering in a future filled with innovative possibilities and transformative trends across various sectors. As advancements in edge computing, sensor technology, and AI algorithms continue to accelerate, the convergence of IoT and AI will reshape industries, enhance services, and revolutionize user experiences. Exploring these emerging trends can illuminate the path forward for organizations seeking to harness the full potential of IoT-AI solutions.
Advancements in Edge Computing
- Distributed Intelligence: Edge computing enables IoT devices to process data locally, closer to the source, reducing latency and enabling real-time decision-making.
- Reduced Latency: By minimizing delays in data processing, edge computing enhances the responsiveness of IoT-AI systems for critical applications.
- Enhanced Security: Performing AI computations at the edge can improve data security and privacy by limiting exposure to external networks.
Proliferation of Intelligent Sensors
- Sensor Fusion: Integrating multiple sensors in IoT devices enables comprehensive data collection and enriched insights for AI analysis.
- Embedded AI: Smart sensors with built-in AI capabilities empower IoT devices to make autonomous decisions and adapt to dynamic environments.
- Localized Processing: IoT sensors equipped with AI can perform localized processing, reducing reliance on centralized cloud resources.
Advancements in AI Algorithms
- Explainable AI: Transparent and interpretable AI algorithms help users understand how decisions are made, fostering trust and accountability.
- Reinforcement Learning: AI systems leverage reinforcement learning to continuously learn and improve performance based on feedback.
- Federated Learning: Distributed training of AI models using federated learning enhances privacy and customization without centralizing data.
Conclusion
In conclusion, the fusion of IoT and AI represents a groundbreaking opportunity for organizations to innovate, optimize, and transform their operations in the digital age. By leveraging the collective strengths of IoT devices for data collection and AI algorithms for analysis and decision-making, businesses can unlock unprecedented insights, efficiencies, and competitive advantages. While challenges persist in terms of interoperability, security, and skills development, the future outlook for IoT-AI integration is undeniably bright, paving the way for a smarter, more connected, and AI-driven world.
In the era of IoT-AI convergence, organizations must prioritize upskilling their workforce, forming collaborative partnerships, and addressing ethical and regulatory concerns to ensure responsible and successful deployment of IoT-AI solutions. By investing in training programs, leveraging external expertise, and adhering to privacy and compliance standards, businesses can navigate the complexities of this transformative technology landscape while maximizing its potential for innovation and progress.